Faculty
Prof. Leong, Tze Yun, PhD, FACMI, MIAHSI
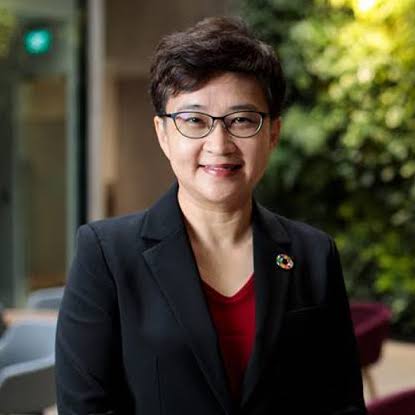
Professor of Practice of Computer Science, School of Computing, NUS
Director, NUSAiL
Advisor, Medical Computing lab
Website
Director, NUSAiL
Advisor, Medical Computing lab
Website
Research staff
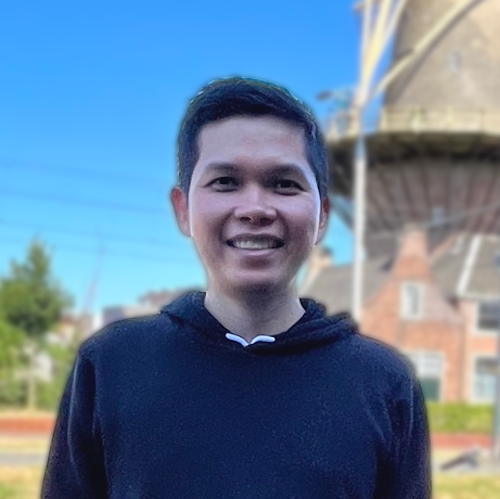
Vo Thanh Vinh
Research Fellow
Causal inference, causal discovery, point processes- Vo, T. V., Bhattacharyya, A., Lee, Y., & Leong, T. Y. (2022). An Adaptive Kernel Approach to Federated Learning of Heterogeneous Causal Effects. Advances in Neural Information Processing Systems, 35, 24459-24473. https://proceedings.neurips.cc/paper_files/paper/2022/file/9a9afa70eead1805f00e3a0df2a41157-Paper-Conference.pdf
- Vo, T. V., Lee, Y., Hoang, T. N., & Leong, T. Y. (2022, August). Bayesian federated estimation of causal effects from observational data. In Uncertainty in Artificial Intelligence (pp. 2024-2034). PMLR. https://proceedings.mlr.press/v180/vo22a/vo22a.pdf
- Vo, T. V., Wei, P., Bergsma, W., & Leong, T. Y. (2021, March). Causal Modeling with Stochastic Confounders. In International Conference on Artificial Intelligence and Statistics (pp. 3025-3033). PMLR. http://proceedings.mlr.press/v130/vinh-vo21a/vinh-vo21a.pdf
- Vo, T. V., Wei, P., Hoang, T. N., & Leong, T. Y. (2022, October). Adaptive Multi-Source Causal Inference from Observational Data. In Proceedings of the 31st ACM International Conference on Information & Knowledge Management (pp. 1975-1985). https://dl.acm.org/doi/pdf/10.1145/3511808.3557230
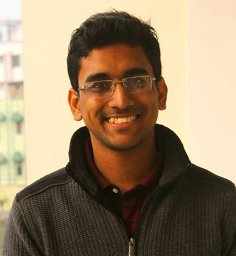
Ambastha Abhinit Kumar
Research Fellow
Incremental Learning, Domain Adaptation, Transfer Learning[Google scholar]
Students
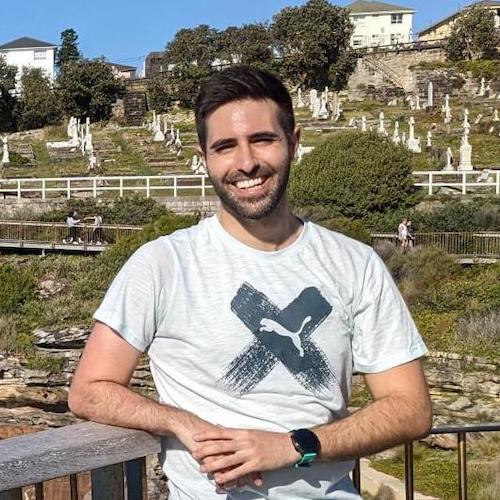
Evangelos Sigalas
Ph.D. Candidate, 2019
Causal Connectivity Inference from Individual Neurons to Multiple Interacting Neuronal Populations in the Brain.I want to understand how brain regions communicate. This is expressed in the effective connectivity between them and studying it can give us an understanding of the problem solving skills of the brain.
[Google scholar]
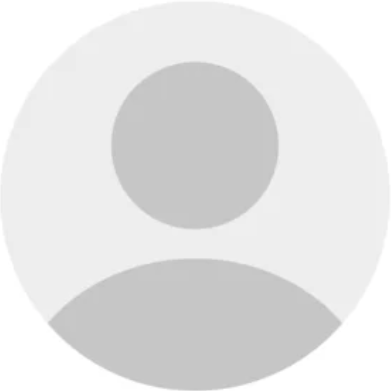
Di Fu
Ph.D. Candidate, 2019
Deep learning, continual learning, incremental learningMy research interest centers on the development of robust and scalable AI algorithms capable of continual learning in the dynamic and ever-changing world.
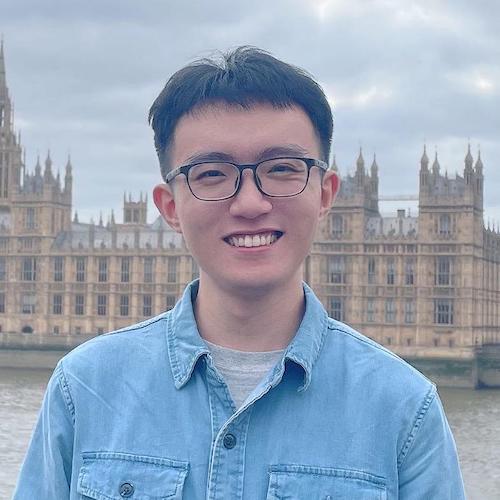
Ma Haozhe
Ph.D. Candidate, 2021
Reinforcement LearningMy research is focused on general reinforcement learning theory, hierarchical reinforcement learning, human-AI collaboration and knowledge based imitation learning.
- Haozhe Ma, Thanh Vinh Vo, and Tze-Yun Leong. 2023. Hierarchical Reinforcement Learning with Human-AI Collaborative Sub-Goals Optimization. In Proceedings of the 2023 International Conference on Autonomous Agents and Multiagent Systems, 2310–2312.
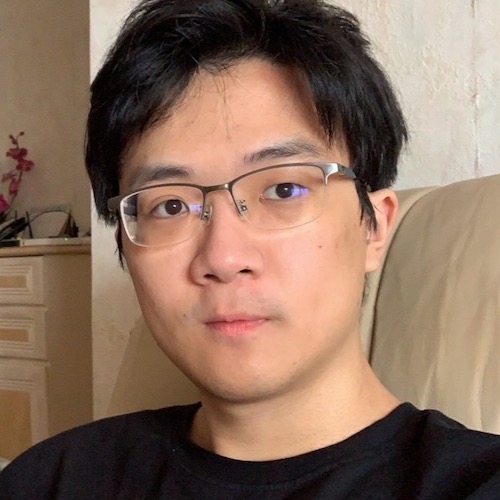
Wu Jiele
Ph.D. Candidate, 2022
Graph neural networkI am currently working on causal representation learning and graph neural network. My research interests are causal representation learning, neuroAI & cognition, graph learning.
- Jiele Wu, Chunhui Zhang, Zheyuan Liu, Erchi Zhang, Steven Wilson, and Chuxu Zhang. GraphBERT: Bridging Graph and Text for Malicious Behavior Detection on Social Media. ICDM 2022 https://ieeexplore.ieee.org/abstract/document/10027673
- Jiele Wu, Chau-Wai Wong, Xinyan Zhao, and Xianpeng Liu.Toward Effective Automated Content Analysis via Crowdsourcing. IEEEInternational Conference on Multimedia and Expo (ICME'21), Shenzhen, China, Jul. 2021, published. https://ieeexplore.ieee.org/abstract/document/9428220
- Wenmeng Yu, Hua Xu, Ziqi Yuan, Jiele Wu. Learning Modality-Specific Representations with Self-Supervised Multi-Task Learning for Multimodal SentimentAnalysis. AAAI 2021 https://ojs.aaai.org/index.php/AAAI/article/view/17289
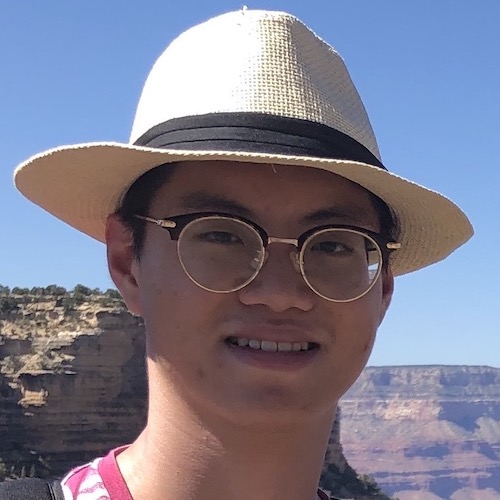
Woo Hao Xuan
Ph.D. Candidate, 2022
Time series modellingI’m currently working on a more accurate way of estimating the variational approximation of the posterior distribution within the variational inference framework. In VI, we want to minimize a lower bound of the marginal likelihood (ELBO). This arises because the exact formulation of the marginal likelihood is intractable. To do so requires the introduction of a variational distribution q(z|x) that approximates p(z|x). We can show that if q approximates p well, then we can minimize the ELBO. The way I’m doing it is using sequential Monte Carlo (particle filters) and ensemble Kalman filter methods to empirically estimate the distribution of q.